Categories
Do you know a Fred?
Fred’s day could be captured as a series of numbers, characters, symbols, images, and sounds.
- 13 is the number of kms he walked from the uptown homeless shelter to the downtown drop-in centre
- 2.2 hours is how long he spent doing laundry at the drop-in
- 90 was his heart rate at the shelter last night
- 4 is the number of beers he’s drunk
- $455 dollars is the debt he owes the storage centre today
- 3 is the number of unanswered Facebook messages from family
- ??? are the emojis Fred uses to describe his mood
- 75 dBA is the average sound of the drop-in centre
- 1 is his motivation level.
Fred’s life could also be captured as a series of numbers, characters, symbols, images, and sounds.
- 7 is the age Fred was removed from his parents
- 6 is the number of failed foster care placements
- 31 are the months he has spent in jail
- 123 are the number of books he read in jail
- Astrophysics is his favorite topic
- 55 is the number of building sites Fred has toiled at
- 60K is how much Fred has claimed in unemployment & long-term disability benefits
- 1020 are the nights he has slept rough
- 7 are the number of services with which he interfaces
- 4 out of 10 is Fred’s optimism about the future
This is data – the “raw material produced by abstracting the world into categories, measures, and other representational forms (Kitchen, 2014).”
And yet for all the possible data about Fred (or people like him), we estimate less than 20% would be usable information. Even less would be useful knowledge.
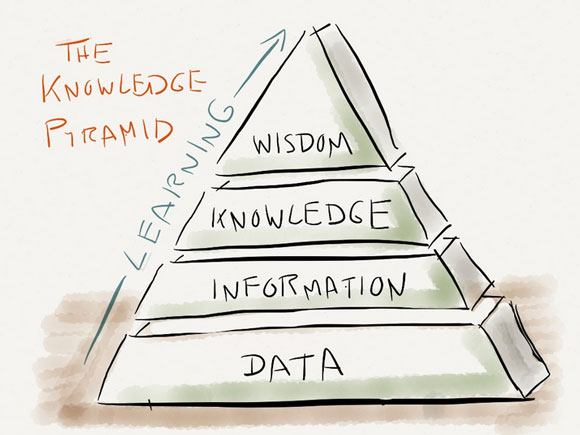
Most of the data is not regularly collected. Of the data that has been captured, the majority languishes in paper files, notebooks, outdated excel spreadsheets, and desktop software systems. Social media and banking are the exceptions. Every time Fred logs into Facebook, his clicks and activities are logged and aggregated within an enormous, dynamic database. The same is true with Fred’s bank account. Every financial transaction – be it a government deposit of $498 or a $200 cash withdrawal – is coded and analyzable.
Where big data are generated continuously and often automatically (think: financial transactions), hard data are generated at a point in time and often manually (think: census). Thick or small data, by contrast, are generated through observation and conversation. What emerges is far more nuanced information, conveying people’s emotional (and not easily reductive) life experiences. Indeed, Fred’s state of mind and why he feels the way he does cannot simply be boiled down to one number. With humanness also comes ambiguity.
Data may be everywhere, but how to collect and for what reasons are very much pressing questions.
In “Big Data is our generation’s civil rights issue and we don’t know it,” the journalist Alastair Croll walks readers through some scarily likely scenarios. Your email is linked to your Amazon basket, to your social media accounts, even to your dating profile. Together, your posts reveal what you might never have self-reported: your gender, ethnicity, and disability status. And since marketers are already accessing this information to personalize the advertisements you see, it’s not too far a step to imagine insurance companies, banks, and employers using this information to deny you coverage, a loan, or a job. In Croll’s words,
“Personalization is another word for discrimination.” But, what if it wasn’t private corporations discriminating against you, but community groups and social services offering you feedback or a menu of opportunities? Would it be OK then?
Peter Manzo, the CEO of United Way Bay Area, wonders how non-profits might proactively engage users, rather than wait for people to knock on their door. He writes,
Might it be more effective to use big data to push resources to people who haven’t sought them out? Opt-in is important ethically, but it also takes work and is a bit of a barrier. If we could get results on a greater scale by pushing messages without an opt-in (the way search engines and social media applications do) would we? Take San Bernardino County in California, where approximately 140,000 people are eligible for food assistance but do not receive it. A supermarket chain or a grocers’ association probably could purchase or collect data from these families to reach out to them, and we wouldn’t criticize them—in fact, we may honor them for it. Shouldn’t a hunger charity be able and willing to do the same?”
Intent clearly matters. Using personalized data to generate feedback for you is different than using such data to penalize or stigmatize you. Using aggregated data to understand service usage trends is different than using such data to decide on where to close services. There are at least three dimensions to purpose worth disentangling:
(1) is the emphasis on understanding or oversight?
(2) is the goal to generate ideas or to justify decisions?
(3) is the focus on an individual or the population?
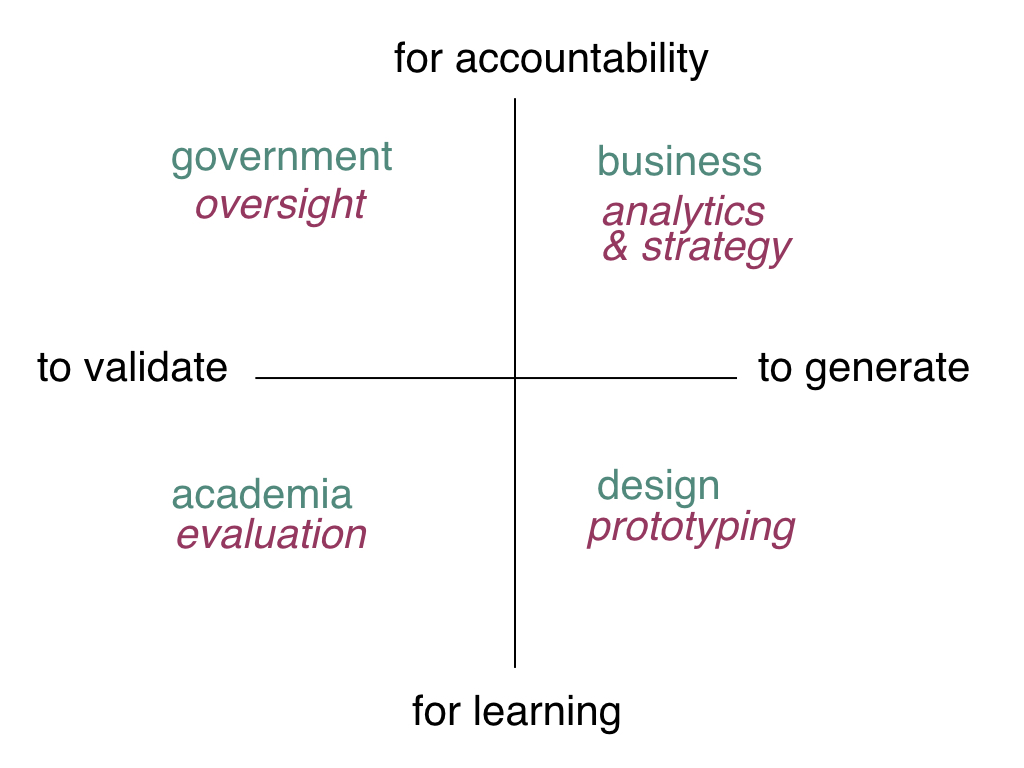
We can broadly map purpose to perspectives. Different stakeholders tend to assume different roles. Where government is often in an oversight role, academics are often in an understanding role. Where service providers are often in justification mode, designers are typically in a generative mode.
What would these different perspectives have to say about Fred?
Designers might use data about Fred’s drinking, moods, sleep and heart rate to help him identify triggers and spark motivation for behavior change. The emphasis might be on data for understanding in order to generate ideas at the individual level. Indeed, there’s plenty of good behaviour science literature pointing to the value of feedback loops in improving motivation, goal orientation, hopefulness, and change. For feedback to be useful, behavioural level data (e.g Fred’s drinking) must be measured and stored. Then, that data must be sent back to the user as information that is relevant and emotionally resonant.
Challenge is, government could use the same individual level behavioural data in an oversight capacity to justify decisions like cutting benefits. From their point of view, why should taxpayer dollars go towards alcohol? (This is not far fetched. In some US states, welfare beneficiaries are prohibited from spending their benefits on alcohol or tobacco). At an aggregated or population level, government might draw on data about people’s longitudinal service usage to justify procurement decisions and hold non-profits to collective account. But, the same data about service patterns over time, might lead a service design team to create a missing category of service.
Herein exposes what makes data inherently political. Data is a raw resource in the same way land, water, and minerals are resources. Who retains ownership and who can can extract and exploit value shifts vulnerabilities and risks in a very real way. How do we ensure people on the margins are not left worse off? Who controls which data is shared with whom? And when do collective safety concerns trump individual agency and choice?
I think the solution to the challenges of informed consent, transparency about methods, and protecting client confidentiality will be found in giving users the ability to know their data is being used, how and by whom, and giving them the ability to withdraw their data from the big data set whenever they like. But we need to work to give them that ability, not foreclose it for them because we fear we can’t be trusted with it.
Trust is the essential basis for resource sharing. Scholars Charlotte Hess and Elinor Ostrom argue that information and knowledge should be conceptualized as a collective resource equivalent to parks or libraries.
[We need] a new way of looking at knowledge as a shared resource, a complex ecosystem that is a commons —a resource shared by a group of people that is subject to social dilemmas…“
This is one of the arguments behind open data. Open data is both a set of values and frameworks. Any flavor of data – big, hard, small or thick – can be made openly available, provided there are clear standards around format, structure, and use. Hess and Ostrom point out that there is a strong empirical base for developing such standards. They note: “One of the truly important findings in the traditional commons research was the identification of design principles of robust, long enduring common pool resource institutions.”
Principles include
- Clearly defined boundaries should be in place.
- Rules in use are well matched to local needs and conditions.
- People affected by the rules can participate in modifying the rules.
- The right of community members to devise their own rules is respected by external authorities.
- A system for self-monitoring members’ behavior has been established.
- A graduated system of sanctions is available
- Community members have access to low cost conflict resolution mechanisms.
The New Zealand Data Commons Project is trying to apply these principles to the social sector. They persuasively argue that while the music, media, retail and hospitality sectors have been totally disrupted by big data, the state and social sectors have barely adapted. Two barriers stand in the way: (1) government acts as a centralized hub with high transaction costs and low information visibility and (2) fragmentation prevents looking at the end-to-end journey of users, their pathways across services, or a picture of their whole lives. They write,
The challenge is not technical. That part has been solved. The real challenge is adapting how we work. The social sector currently operates under a centrally-controlled model that has divided the sector into competing self-interests, orientated towards the centre…To realize its latent influence, the social sector needs to build trust, share those data assets, and act in the common interest.
This is why the Data Commons Project is adopting a universalist, protocol-based approach. Members of the data commons will be able to sign-up to a set of specific principles, protocols, and use cases which are co-designed and co-governed. Practically that means each organization agrees to build their tools and technologies in such a way that they can talk with one another with clearly articulated intent. Data will be linked with how it can be used, by whom. Data sharing agreements will be transparent.
So, what would a Data Commons Project look like for our collective work? What might be our specific principles, protocols, and use cases? And what would they do for Fred and the services which support him?